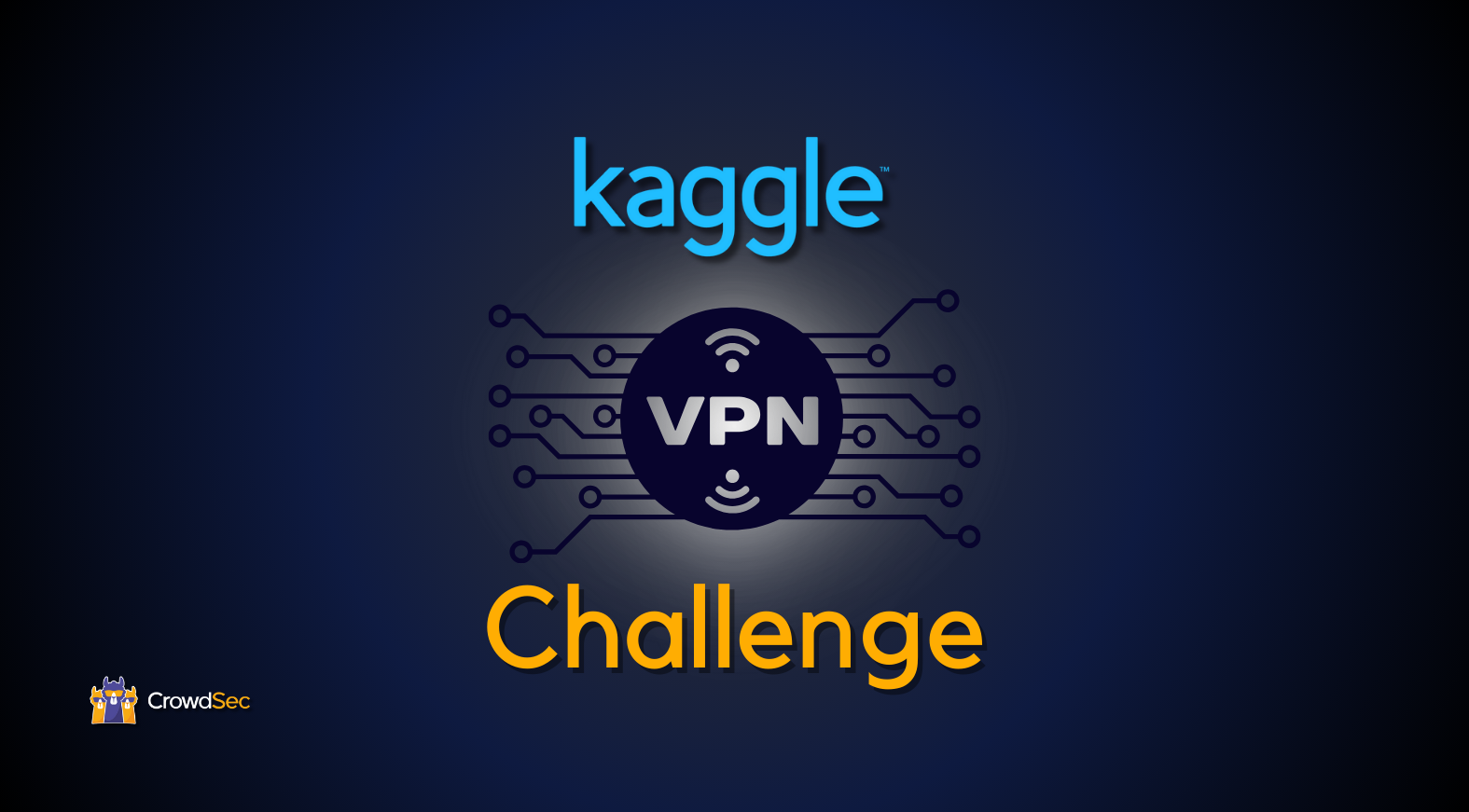
Detecting VPN and Proxy Usage via IP Traffic Analysis: A Glimpse into CrowdSec's Kaggle Challenge
In an era where digital threats are constantly evolving, the need for innovative approaches in cybersecurity has never been more pressing. At CrowdSec, we sincerely believe in the power of the crowd in the field of collective cybersecurity. In addition to our free and open source software, we redistribute the most active malicious IPs reported by the community to our users in real time.
Enhancing cybersecurity through collective intelligence
This ethos of collective defense is the driving force of our latest initiative — a Kaggle community competition that invited everyone to tackle a pressing issue in cybersecurity: the detection of VPN and Proxy traffic.
We provided participants with 60 million attack signals crowdsourced from our Security Engine users over a one-month period. A complementary dataset was attached, representing the metadata of each compromised machine extracted from Shodan, containing descriptions of ports, services, and several connection hashes.
During 3 months, between October and December 2023, 85 participants competed with their best analysis and modeling skills to identify compromised machines that were used as VPN or proxy services. More than 1000 submissions were sent on the platform! That's an incredible level of participation we never imagined reaching when we launched this challenge. The most exciting moment was dissecting the winning candidates' solutions and analyzing the key takeaways.
And now, it’s time to share the findings and most interesting highlights of our Kaggle challenge with the world — we hope you find them as fascinating as we did!
Detecting VPN and proxy usage via IP traffic analysis: Key takeaways
Shodan thrills
As in any Kaggle competition, feature engineering is an angular stone that relies on most of your model's performance. The first and most creative feature we want to outline is the use of the metadata displayed in the Shodan data. The headers hash and the fingerprinting tags (JARM and JA3M) are crucial to identifying a VPN or a proxy.
It’s very intuitive; if several users share an IP address, it will display many services and open ports. Simple ways of exploiting this can be counting the number of TCP and UDP services listening on a compromised machine or transforming the most used port numbers into one-hot-encoding features.
But most importantly, since different users can use the same services, we saw a high amount of hash and fingerprints being used by the same IP. This feature was proven to play a significant role in high-rank models.
One exciting part is how to handle the JARM signature by cutting the first 30 characters. As specified in the JARM documentation, “if the first 30 characters are the same but the last 32 are different, this would mean that the servers have very similar configurations, accepting the same versions and ciphers, though not exactly the same given the extensions are different.”
For this reason, many IP addresses sharing the same first 30 characters of the JARM means that similar servers are running behind the IP address. This can be an example of a single machine switching between several VPN/proxy, or similar machines compromised and operated by the same entity.
Temporality is Key
Another important feature is the evolution of the attack pattern for a given compromised asset over time. It is possible to extract a time series of all the alerts reported for an IP address from the signals dataset. An IP address being reported for a wide range of attacks consistently over time, is likely a sign that multiple machines use the same IP address. The most reliable features for this can be built using a rolling window to compute the number of attack_type evolutions.
Your neighborhood tells a lot about yourself
Looking at the reports from machines hosted on the same IP range reveals much information about an IP. This information was no secret, as indicated in our last Majority Report. Some ranges are specialized in hammering the SSH port and trying to brute force servers. For other services like VPN or proxy, it is expected to manage an entire IP range or Automation System — except for the residential proxy.
In such a situation, it makes sense to look at the number of VPNs flagged in the same area and use this count as a feature in our model. This valuable information will be soon featured in Crowdsec Threat Intelligence Search.
Similarly, one can also look at the popularity of hash and header, which can be reused across different IP addresses when switching IP addresses.
Conclusion: A step towards a safer digital future
On behalf of the CrowdSec Data and Core Teach teams, I want to personally thank all the participants in the competition; there were a lot of innovative ideas that will inspire us to build the next version of the algorithm. Sharing with the community and getting feedback is a virtuous circle that makes this journey so delightful.
Congratulations to all the winners for their performance and a special shout out to André Minoro Fusioka for reaching first place and winning a GPU RTX 4080!
This competition was also a springboard for us to notice new talents to join the CrowdSec workforce. And for that, a special shout-out goes to Frederico Santos, who had to withdraw from the competition despite being ranked among the top tier because he was recruited to the CrowdSec team.
Stay tuned because we will most certainly come back with other crunchy topics in the area of anomaly detection for our new AppSec Component!