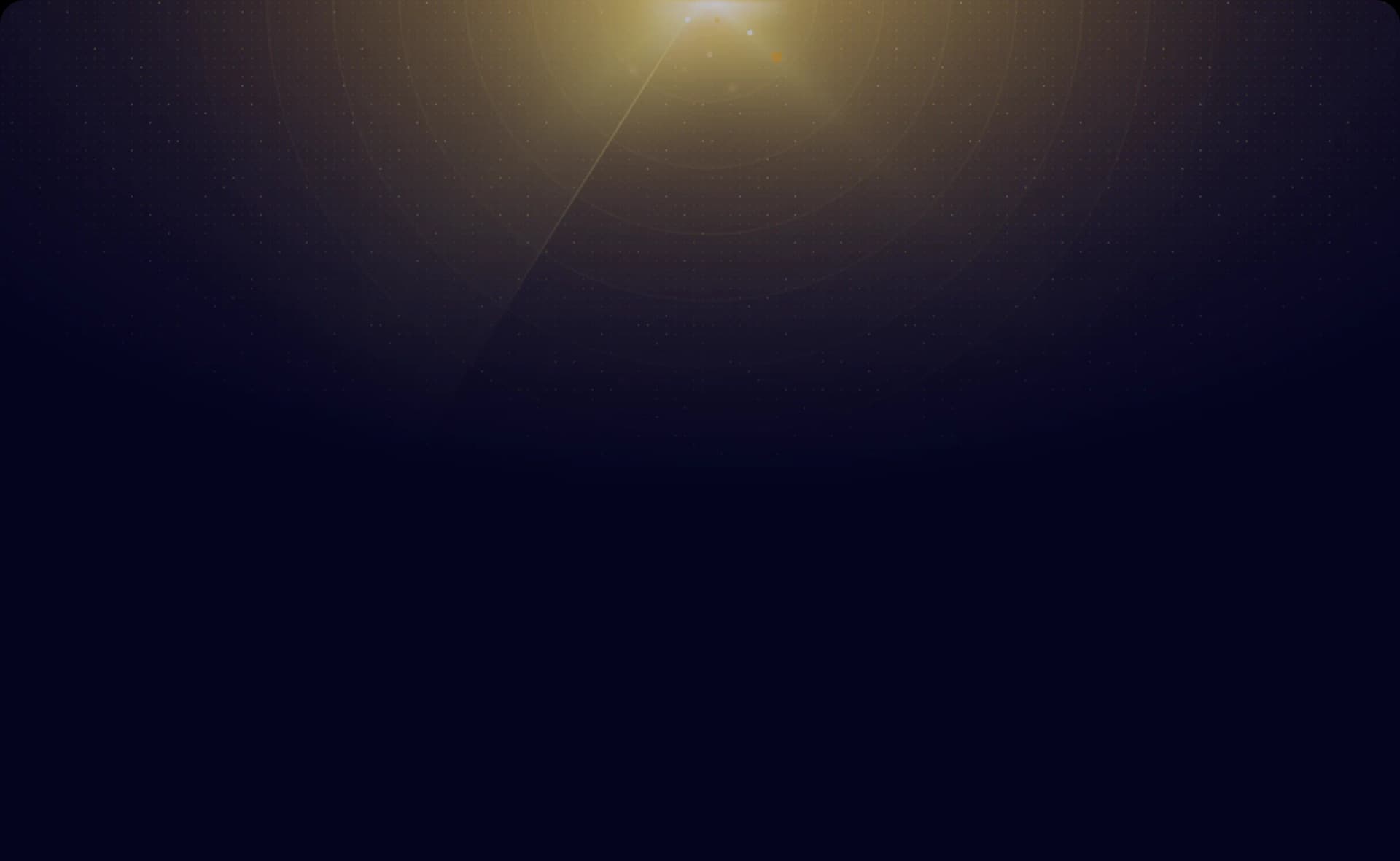
The CrowdSec Data
Explore CrowdSec’s Fail-Proof Approach to Tactical Intelligence
Frequent updates
Crowd-powered insights
Ultra-curated data
say goodbye to:
Overblocking IPs
Lack of VPN/Residential Proxy Detection
False Positives
1. Philosophy and Vision
The reason we chose the collaborative approach is simple. Solely relying on a honeypot network could never yield the results needed for building a global CTI. Honeypots are expensive to run, and the services to which they are exposed are not as trustworthy as a real machine. Running a fake WordPress server with low SEO will only catch very spammy attackers. On the other hand, having access to real machines with thousands of visitors, hosting content of interest, and reporting real targeted attacks, is what makes the difference between an average CTI and a top-quality CTI.
CrowdSec embraces a unique approach to cyber intelligence by leveraging the power of the crowd to provide an open source and collaborative method for data collection. This is what we call, The Network Effect of Cyber Threat Intelligence.
2. Data Collection
2a. How It Works
The open source components parse logs to detect an attack behavior. If an attack pattern is recognized, it will raise an alert and optionally remediate the attack locally. The collaborative part of the Security Engine aims at building a global CTI network crowdsourced by the community — i.e., the installations of Security Engines all over the world.
When an attack is detected locally, the users can choose to share the information with the community. In return, the users benefit from information shared by other community members, which leads to stronger protection, as attackers are banned before their attacks are perpetrated.
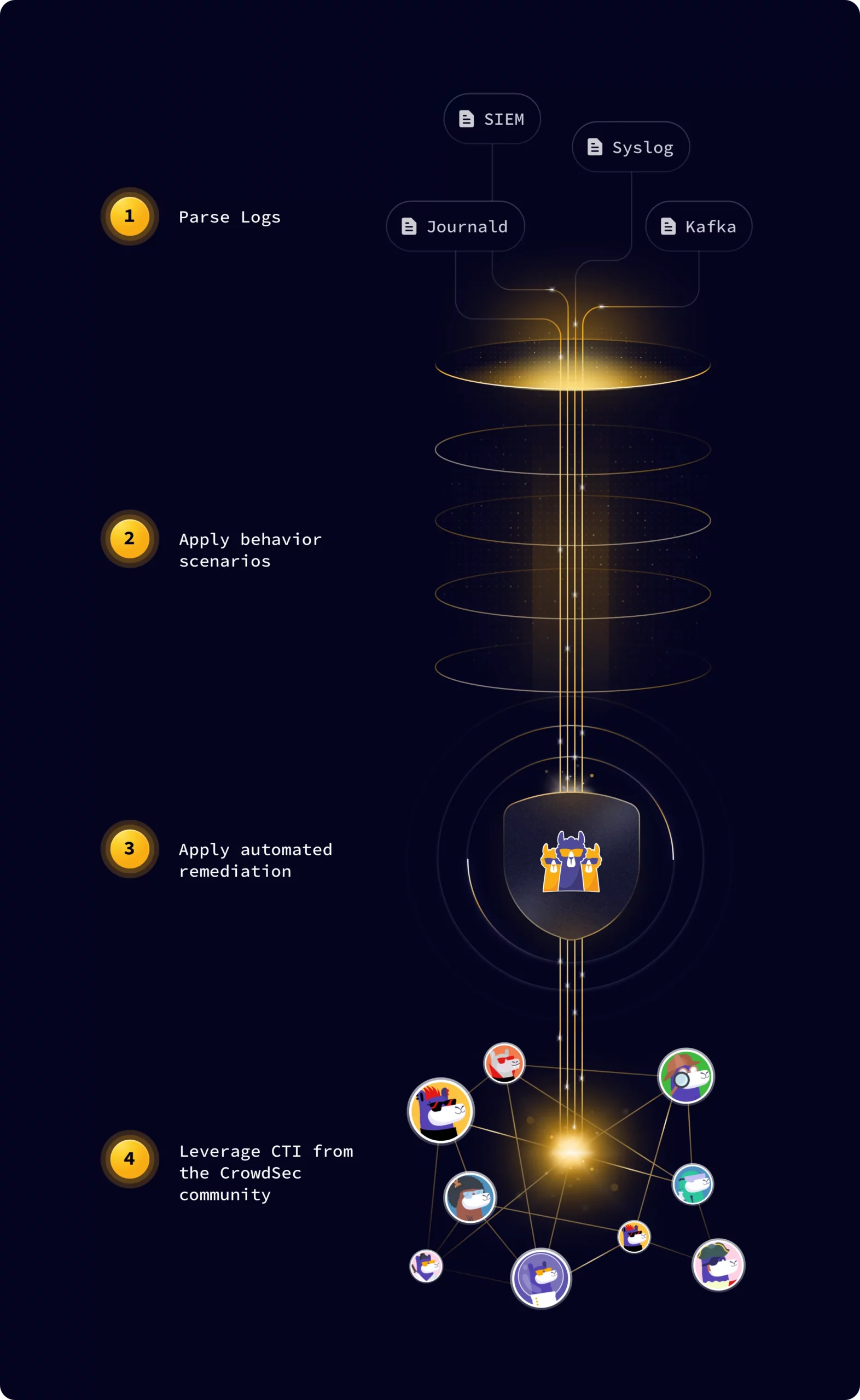
2b. The Concept of Consensus within the CrowdSec Network
We refer to the term consensus to describe the aggregation algorithm that builds the community blocklist based on the crowdsourced IP addresses. The consensus algorithm determines whether an IP address is malicious at any given moment and when it ceases to be malicious. Its task is to discover the truth in the crowdsourced threat intelligence data and redistribute the most relevant attackers in a timely manner.
3. Overcoming the Challenges of Redistributing Crowdsourced Data
The CrowdSec team is working hard to ensure the accuracy of our data and we are continuously looking for ways to improve our data collection, analysis, and curation methods to overcome certain challenges that inevitably come with redistributing crowdsourced data.
There are three major challenges to overcome:
- Confirm an IP is indeed malicious
- Prevent false positives from system misconfiguration
- Prevent false positives from data poisoning
Being resilient to data poisoning is a necessary condition for the efficiency of the network. In addition, we must also evaluate the quality of the information reported to limit the number of errors. For this purpose, we designed a Trust Scoring System to evaluate the reliability of our contributing users. This Trust Scoring System relies on several criteria, which are defined below. The level of the threat is then evaluated by compounding for each Indicator of Compromise (IoC), the number of signals reported with the trust score computed previously, and the nature of the attacks reported.
4. Trust Scoring System
For every scenario and user reporting a signal of a malicious IP, there is a trust score which is computed — the Scenario Trust Score and the User Trust Score.
💡 Scenarios consist of rules that define patterns of behavior indicative of a security threat. As an example, when detecting brute force attacks, the detection scenario would look for repeated failed connection attempts within the logs.
4a. Scenario Trust Score
Spoofability
Measures how easy it is for an attacker to hide their IP. A scenario might correctly detect an attack, but the attacker might be able to spoof the source IP that will appear in the logs — for example, when logging the source IP of UDP packets at the firewall level.
Confidence
Measures how certain we are that the attacker was actually being malicious. System misconfiguration configuration on the part of the user could potentially cause a normal visitor to trigger an action that is interpreted by the Security Engine as an attack.
This score ranges from 0 to 3 and helps to weigh the number of signals for each scenario. If the confidence level is 3, the maximum, 100% of the signals are kept, and if the level is 0, the signals are discarded entirely. For level 1, we keep 25%, and for level 2, we keep 50%.
4b. User Trust Score
For the User Trust Score, there are various criteria taken into consideration.
i. User Commitment
User Longevity
The user’s longevity represents the longest uninterrupted period of time during which they reported an IP and interacted with our central API on a regular basis. User Longevity, or activity period, while not proof of the user’s intention, shows a commitment to the project in the sense that the user is dedicating certain resources (CPU, memory, bandwidth, etc.) to running the Security Engine and thus increasing the cost for an attacker.
ii. Report Consistency
Assessing the quality and relevance of information submitted by users by cross-checking signals on malicious IPs shared with trustable sources of information. Naturally, users sharing inconsistent information get a lower trust score.
To prevent people using rented or breached machines from poisoning the consensus mechanism (e.g., a rented botnet), we evaluate the consistency of reports and the likelihood of the reports coming from known malicious actors. To evaluate this, we primarily rely on three sources.
Honeypots
CrowdSec is running a network of honeypots spread across various public clouds, as well as partners who allow us to run honeypots within their network. The honeypots themselves are running Security Engines and are exposing various services or simulated services. The reports coming from those honeypots are considered a source of truth, given that we carefully choose scenarios that are not subject to spoofing.
Anomaly Detection
We cross-check reports to make sure that the user reporting an IP has not been reported by another user, which would increase the chances of the IP reported being a false positive. If this occurs, we diminish the user’s trust score.
iii. Honeypot Overlap
When it comes to relying on honeypot information to evaluate the veracity of user reports, time is key. Given that honeypots are a source of truth, we can safely say that an IP reported for a given behavior by a user and then, later on, reported by a honeypot for the same kind of attack, shows that the user is very likely running real services exposed on the internet.
5. Evaluating Treats and Aggregating Scores
The attackers now essentially have to pass through two filters.
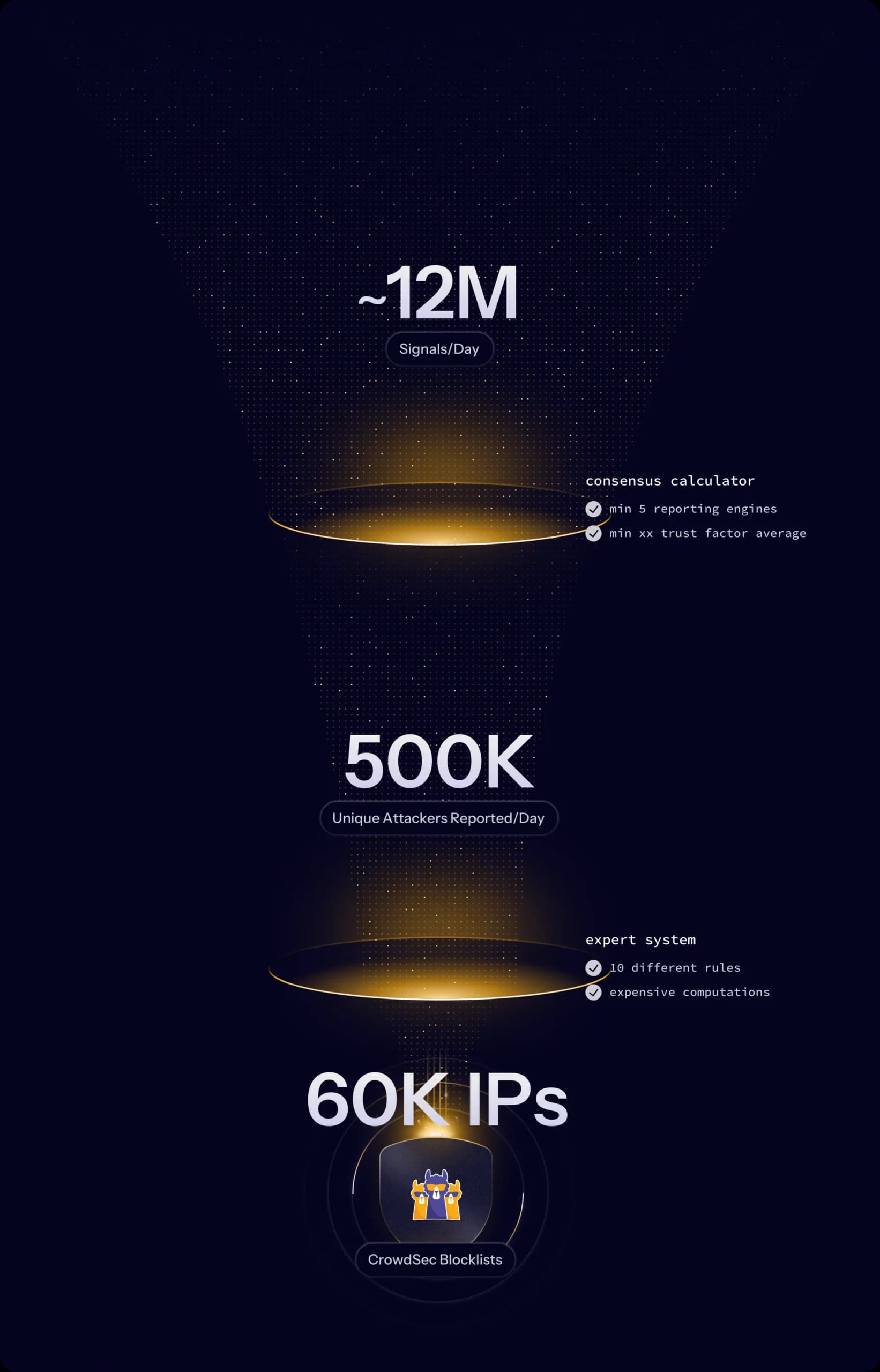
Consensus Calculator
Among other things, the Consensus Calculator acts as a filter for attackers. The majority of the attacks that the CrowdSec Network sees are isolated attackers who attack one or two machines before vanishing. These attackers are dangerous to the individual user, and the Security Engine protects its users against them. However, there is no point in adding those isolated attackers to the blocklist that goes out to all our users as the attacks are not relevant to them. So, to avoid redistributing irrelevant IoCs with our community blocklist, the Consensus Calculator filters out attackers that do not pass a minimum baseline in the number of reports and the trust score of the reporting users. For attackers that pass these baseline rules, it then calculates additional metrics, which are fed to the next step, the Expert System.
Expert System
The Expert System is a purpose-built rule engine that evaluates the attackers in detail. It has many different rules that encode different reasons why an attacker might be considered threatening enough to end up in the community blocklist. There is a basic rule that considers simple factors such as a minimum level of trusted reports and diversity criteria that guarantee the IP is reported from a high number of users, IP ranges, and Autonomous Systems. There are also more specialized rules that incorporate external information about the attacker — for instance, if it is evident that the machine has a high probability of being infected because it uses an old OS. Other rules are concerned with internal information we have about the attacker, such as whether they have been in the community blocklist in the past or whether their IP range has historically had a lot of its IPs in the community blocklist.
6. Validating Results
Our rules are continuously being improved over time as we develop expertise and new vulnerabilities are discovered. To accommodate this, we have an institutional process that forces us to constantly evaluate our rules. This process includes a recurring tech meeting called Human In the Loop, where our Data Science and Core Tech teams take a look at IPs validated and rejected by the Expert System and decide on actions if the results of the Expert System disagree with our subjective assessment of individual threats. This process allows us to spot mistakes and bugs in a timely manner and continually improve our automated consensus process.
The Expert System is also constructed in a way that allows this tight feedback loop to function. New rules can be put in a shadow mode, where they are not yet used to actually label IPs but are constantly evaluated as part of the Expert System, allowing us to examine the results during Human In the Loop. We also have the capability to simulate and replay the expert system with different rulesets in case of changes or data poisoning attempts.
Evaluating user commitment to the project in terms of data shared, infrastructure cost involved, or proving the ownership of known public infrastructure. We want to prevent attackers from being able to poison consensus cheaply, so people with low commitment get a lower trust score.
CrowdSec uses the Security Engine as our data collection machine. The Security Engine is an open source Intrusion Detection System (IDS) and Intrusion Prevention System (IPS) under MIT license, backed by a community information network.
It is important to note that only scenarios that have been integrated into the CrowdSec Hub and reviewed by the members of the CrowdSec Network have the possibility to influence the community blocklist. For each of the scenarios, we assign a trust score which is computed based on the properties of the scenario.
Weighing the factors of assessing an individual report — split into a trust rating of the reported behavior and a trust rating of the reporter — was the first step. In the next step, we aggregate collected data and use these weighted individual reports at the attacker level.